
EXPERIENCE
Philadelphia, PA
2023-present
Wharton School, University of Pennsylvania
Senior Analytics Fellow and professor
-
Oversee multiple simultaneous analytics consulting projects
-
Conduct research
-
Develop data science content for broad dissemination
-
Teach analytic courses
Philadelphia, PA
2008-2023
Director, MS Statistics & Data Science
Director, MS Business Analytics
and professor
Temple University, Fox School of Business
-
Designed data science curricula and courses, including machine learning, visualization, experimental design, data reduction, text analytics, and other state-of-the-art techniques.
-
Designed and launched 3 data science graduate programs.
-
Elected to oversee a committee to redesign the curriculum for the undergraduate program for the school, affecting ≈7,000 undergraduates.
-
Conducted cutting edge research on judgement and decision making in complex environments. Managed every stage of research: design, method selection, data collection, analysis, and presentation of results.
Ithaca, NY
2002-2008
Cornell University, Johnson School of Management
Assistant Professor, MBA Program
Professor in the MBA program. Taught strategic marketing, developed curricula, and conducted cutting edge research.
​
Primary research focus: investigating the psychological processes that underlie decision making and the development of expertise. Research interests include:
-
Human judgment and decision-making
-
Decision support systems, decision aids, debiasing methods, and policy
-
Learning and the development of expertise
-
Quantitative and statistical methods associated with modeling research data
Philadelphia / Ithaca
1997 - present
Data Science / Analytics / Statistics
Consulting
Consultant / Advisor
-
Provided strategic / data science / analytic consulting and knowledge development to 20+ clients in Tech, Financial Services, Real Estate, Telecom, Hospitality, and Publishing.
Washington, DC
1993-1997
Oliver Wyman (Mercer Management Consulting)
Associate
Strategic management consultant to Fortune 500 firms. Industry expertise in financial services and telecommunications; functional expertise in management of technology and marketing research.
> Three-time consecutive recipient of Outstanding Team Award
> Supervised 1-4 consultants and teams of 8-12 client employees
EDUCATION
1997-2002
Philadelphia, PA
Wharton School, University of Pennsylvania
Ph.D., Managerial Science & Applied Economics (Mktg)
M.A., Statistics
Researched the psychological underpinnings of decision making and learning and the statistical and analytic techniques necessary to model complex human behaviors.
1988-1993
Philadelphia, PA
University of Pennsylvania
Jerome Fisher Management and Technology Dual Degree Program
The Wharton School, B.S. Economics
School of Engineering, B.S. Engineering, Computer Systems
TRANSLATE FROM GEEK TO PROFIT
“The current search for people with deep analytical skills is just the tip of the iceberg – more valuable are those who can make business sense of the data.”
– Information Age
SELECTED SKILLS
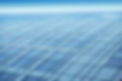
Strategic Analysis / Planning
Financial Analysis
CXO-level Presentation
Complex Project Management
Marketing
Data Science / Statistics / Analytics
Marketing Research
Design of Experiments
Data Visualization
Machine Learning / AI
EXPERTISE
RESEARCH
Experienced researcher with an complete understanding of the process of conducting research from design, data gathering and the organizational ability to manage a project from conception to presentation.
ANALYTICS
Reports no longer sit on bookshelves, but many are incomprehensible or unread. Translation from "geek-speak" to real business outcomes is critical. From data to profit is a mantra, and only a rare few can translate between worlds.
STRATEGY
Superior strategy requires outstanding execution. Complex project management, persuasive skill, integrative teamwork, profit-driven analytics, and data-driven-decision making all need to come together to produce outstanding outcomes.